The market for recommendation engines is projected to grow from USD 1.14B in 2018 to 12.03B by 2025 with a CAGR of 32.39%, for the forecasted period. These figures are an indication of the growing emphasis on customer experience while also being a byproduct of the widespread proliferation of data.
On that note, here are five practical use cases of recommendation systems across different industry verticals. Use these to understand the multiple ways in which recommendation systems can add value to your business.
Five Practical Use Cases of Recommendation Systems
1. eCommerce Recommendations
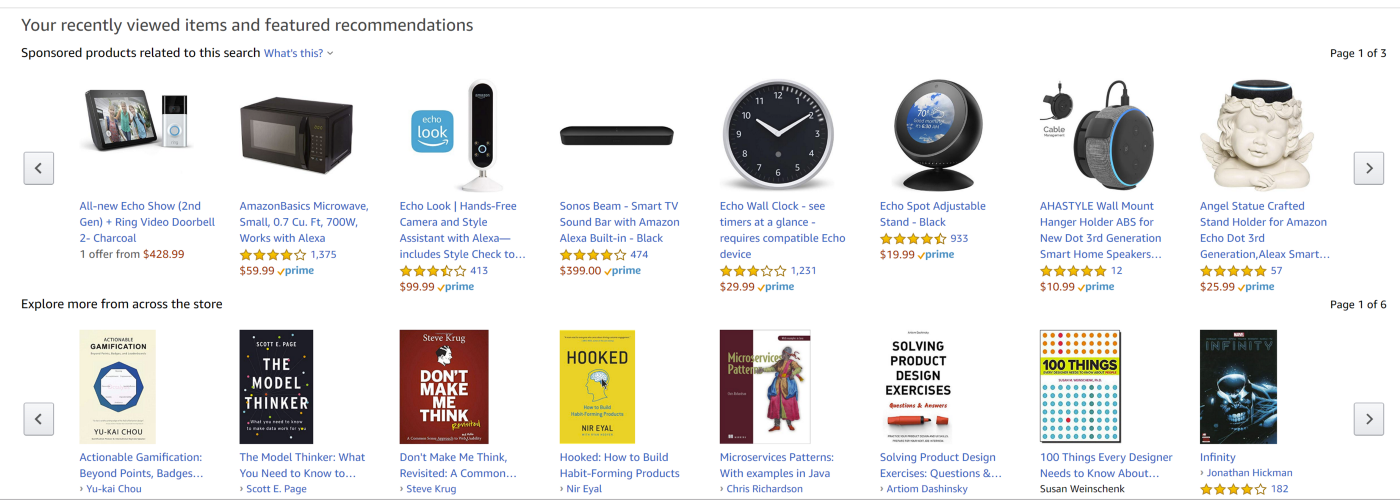
eCommerce is by far the commonest and most frequently encountered use case of recommendation systems in action.
Amazon was a pioneer in introducing this change back in 2012 by making use of item-item collaborative filtering to recommend products to the buyers. The result? A resounding 29% uplift in sales in comparison to the performance in the previous quarter! Soon enough, the recommendation engine contributed to 35% of purchases made on the platform, which was bound to impact the bottom line of the eCommerce giant.
To this date, Amazon continues to remain a market leader by virtue of its helpful and user-friendly recommender engine that now also extends to the streaming platform - Amazon Prime (more on this later). The recommendation system is designed to intuitively understand and predict account interest and behaviors to drive purchases, boost engagement, increase cart volume, up-sell and cross-sell, and prevent cart abandonment.
Other retailers like ASOS, Pandora, and H&M utilize recommender systems to achieve a gamut of favorable results.
2. Media Recommendations

If Amazon is a frontrunner in the recommendation engine race, platforms like Netflix, Spotify, Prime Video, YouTube, and Disney+ are consolidating the role of recommendations in the field of media, entertainment, publishing, etc. Such channels have successfully normalized recommendation systems in the real world.
Typically, most media streaming service providers employ a relational understanding of the type of content consumed by the user to suggest fresh content accordingly. Additionally, the self-learning and self-training aspect of AI in recommendation engines improves relevancy to maintain high levels of engagement while preventing customer churn.
Consider Netflix, for example. About 75% of what users watch on Netflix is a result of its product recommendation algorithm. As a result, it is unsurprising that the platform has pegged its personalized recommendation engine at a whopping USD 1B per year as it maintains sustained subscription rates and delivers an impressive ROI that the company can redirect in fresh content creation.
3. Video Games and Stores
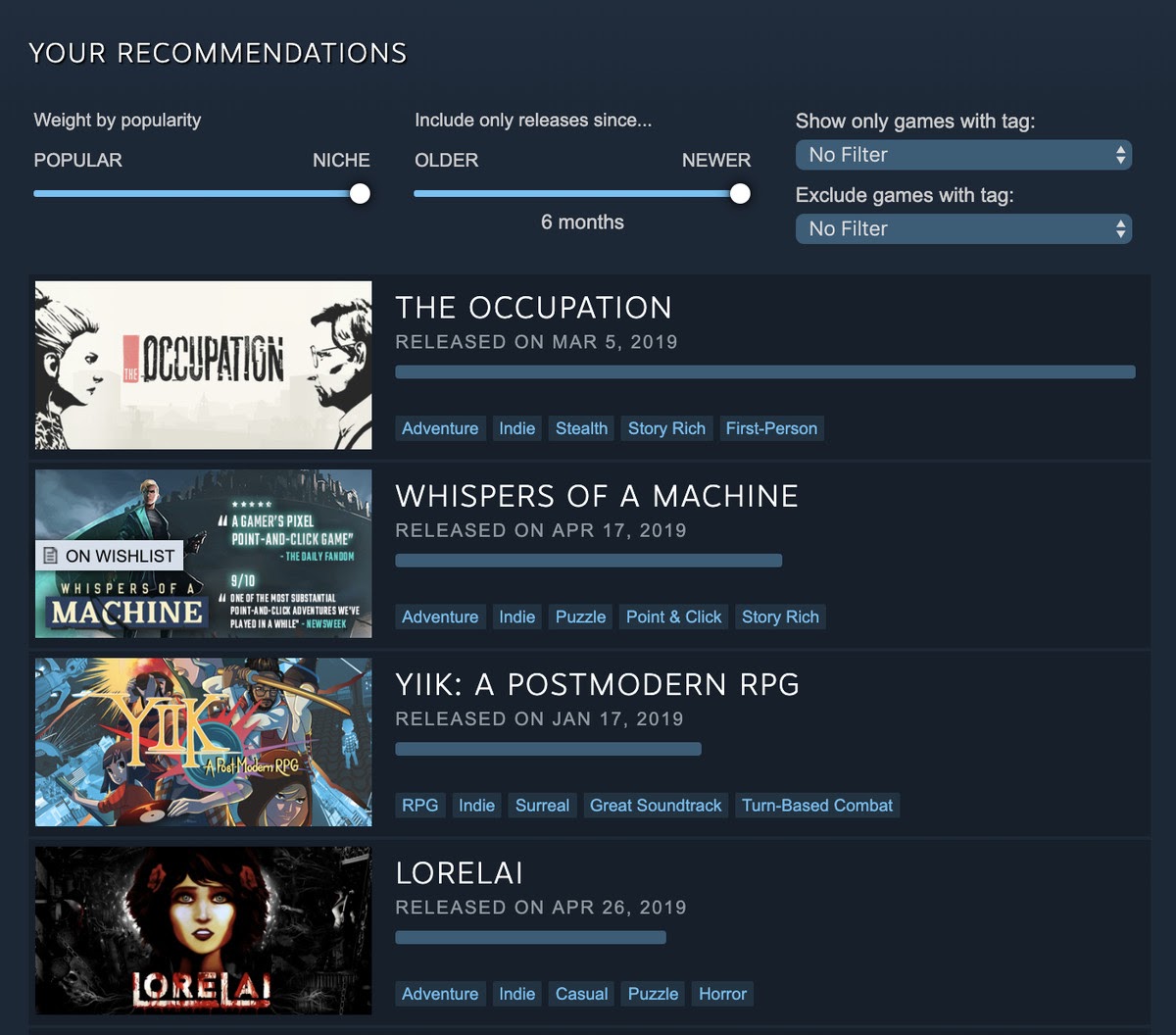
Video games are a treasure trove of user-generated data as it contains everything - from the games they play to the choices they make. This stored repository of action, reaction, and behavior translates into usable data that allows developers to curate the experience to maximize revenue without coming off as pushy or annoying.
Gaming platforms like Steam, Xbox Games Store, PlayStation Store are already well-known for their excellent recommender engines that suggest games based on the player’s gaming history, browsing history, and purchase history. As such, someone who has an interest in battle royale games like Fortnite will recommend games like PUBG, Apex Legends, and CoD rather than MMORPGs like WoW.
Similarly, video games can also deploy recommender systems to nudge players towards the top of the micro-transactions funnel to make the gaming experience easier or more rewarding. Apart from boosting engagements and in-game purchases, AI-based recommender algorithms can unlock cross-selling and up-selling opportunities.
4. Location-Based Recommendations

Geographic location can be a demographic factor that acts as a glue between the online and offline customer experience. It can augment marketing, advertising, and sales efforts to improve overall profitability. As a result, businesses have been in the works of developing a reliable location-based recommender system (LBRS) or a location-aware recommender system (LARS) for quite a while now, and have registered successful results.
Sephora, for instance, issues geo-triggered app notifications alerting customers on existing promos and offers when they are in the vicinity of a physical store. The Starbucks app also follows a similar system for recommending happy hours and store locations. An extension of this feature is seen in Foursquare, a local search-and-discovery mobile app, which matches users with establishments like local eateries, breweries, or activity centers, based on customer location and preferences. In the process, it maintains high engagement levels and promotes businesses in the same breath.
5. Health and Fitness

Health and fitness is one of the newest entrants in the recommender system but enjoys immense potential because of this lag. Applications can capture user inputs, such as their dietary preferences, activity levels, fitness goals, height and weight, BMI, etc., to suggest customized diet plans, recipes, or workout routines to match their fitness goals.
In addition to logging data on such platforms, its integration with wearable devices can streamline its ability to make more accurate and valuable suggestions - such as suggesting meditation or mindfulness exercises to high-risk groups upon registering elevated vitals.
Most importantly, it can capture user feedback on their fitness journey and experiences in the form of ratings to fine tune the plan and make the recommendation smarter and more personalized. Say, someone is dissatisfied with the level of difficulty of the exercise regimen, then the app can recalibrate it to suit their abilities.
Final Words
Recommendations have become an implicit customer expectation as they no longer wish to sift through stores and websites or find things that they do not like. As a result, AI-based content recommendation engines, such as the one developed by Argoid, are no longer a “nice-to-have” feature but the life blood for your business. Talk to us to know more!
FAQs on Recommendation System Use Cases
What is a recommendation system?
A recommendation system uses the process of information filtering to predict the products a user will like and accordingly rate the products based on users' preferences. A recommender system easily highlights the most relevant products to the users and ensures faster conversion.
Why recommendation systems are essential for eCommerce stores?
A recommendation system makes the shopping journey simpler and enjoyable for users. With a recommendation system, you can get product recommendations with minimum search efforts and create long-term engagement.
Which recommendation system should you use?
A recommendation system that you must use is - Argoid. It helps with 1:1 personalized product recommendations for your eCommerce store and ensures faster conversion and retention.