Recommendation engines are driving innovation and agility--in terms of how brands connect with users and how customers experience brands. For billion-dollar brands like Netflix, Alibaba, Amazon, etc., AI recommendation engines have been instrumental in boosting growth and driving organic success.
In this blog, we'll decode what recommendation engines are, how they work, and how they benefit brands to be universally relevant to every user today.
What is an AI-based Recommendation Engine?
A recommendation engine can be thought of as a tool/technology/digital platform that:
- Uses multiple data touch-points such as user preferences, transaction history, attributes, user intent, etc. to deliver a unique and personalized customer experience
- Empowers marketers to offer relevant and personalized recommendations in real-time
- Leverages data filtering tools, algorithms, machine learning, and data analysis techniques to stimulate demand and engage users
The takeaway: Recommendation engines have transformed from being mere data-driven tools to being an engine for innovation, insight, and engagement (pun intended). They are revolutionizing the predictive analytics space to change the way people perceive and experience a brand.
What are the types of AI-driven Recommendation Engines?
Let's look at the three foundational types of recommendation engines, namely:
1. Collaborative Filtering
- There are two types of collaborative filtering techniques: User-User collaborative filtering and Item-Item collaborative filtering.
- This method uses data based on user behavior, preferences, activities, etc., and deploys predictive machine learning techniques to recommend relatable suggestions to users.
- The end goal is to analyze what the users will like based on similar behavior of other users and provide recommendations.
- Its biggest advantage is that it can recommend complex items without gaining an understanding of the item itself.
For example, Amazon uses item-to-item collaborative filtering to provide high-quality recommendations in real-time:
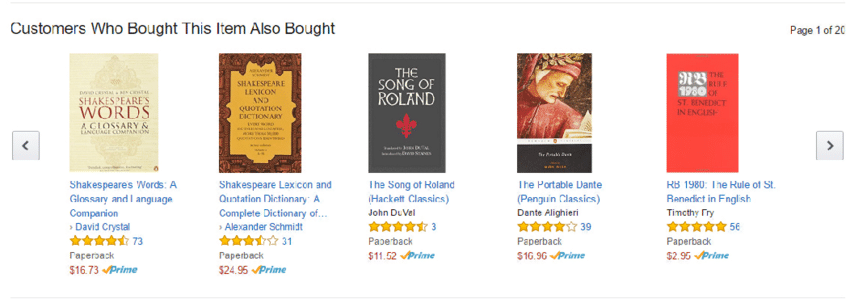
This method matches each user’s purchase history and rated items to similar items so as to provide a recommendation list for the user, thus paving the way for a hyper-personalized shopping experience. In fact, data claims that 35% of Amazon purchases are attributed to recommendation systems.
2. Content-based Filtering
- It may be based on the description of an item, the characteristics of the product itself, or even the appearance of the product which is especially important in the fashion domain.
- This method recommends other products that have similar attributes instead of relying on other users to interact with the product.
- In this method, keywords are used to describe the items.
An example of content-based recommendation would be Stitch Fix’s fashion box which uses machine learning to build an outfit for the user based on their personal style. The brand collects a user’s attributes (think: height, weight, etc.) and matches its fashion products, which are then put in a box and delivered to the user at their doorstep:
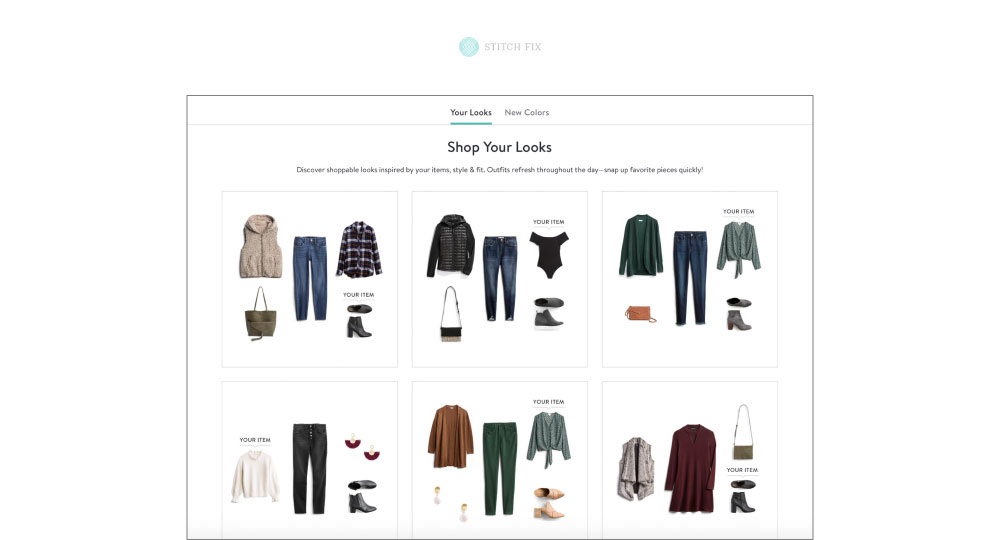
3. Hybrid Model
- It is a combination of diverse rating and sorting algorithms--be it collaborative filtering, product-based filtering, etc. to recommend diverse products to users with greater precision.
- It looks at the collaborative data as well as the content-based data, making it a more optimized option.
Netflix is one of the best examples of a hybrid AI-based content recommendation system. The brand leverages the collaborative filtering method by comparing the browsing and viewing habits of users to find similar users on the platform. Plus, it makes use of content-based filtering to recommend shows/movies which share common characteristics with the ones that were highly rated by the user.
Also according to the brand's website, the data analysis team goes beyond validating the ideas on historical data by running online A/B tests and measuring long-term satisfaction metrics. These experiments allow the brand to move beyond rating prediction, and into personalized ranking, page generation, search, image selection, messaging, and so on.
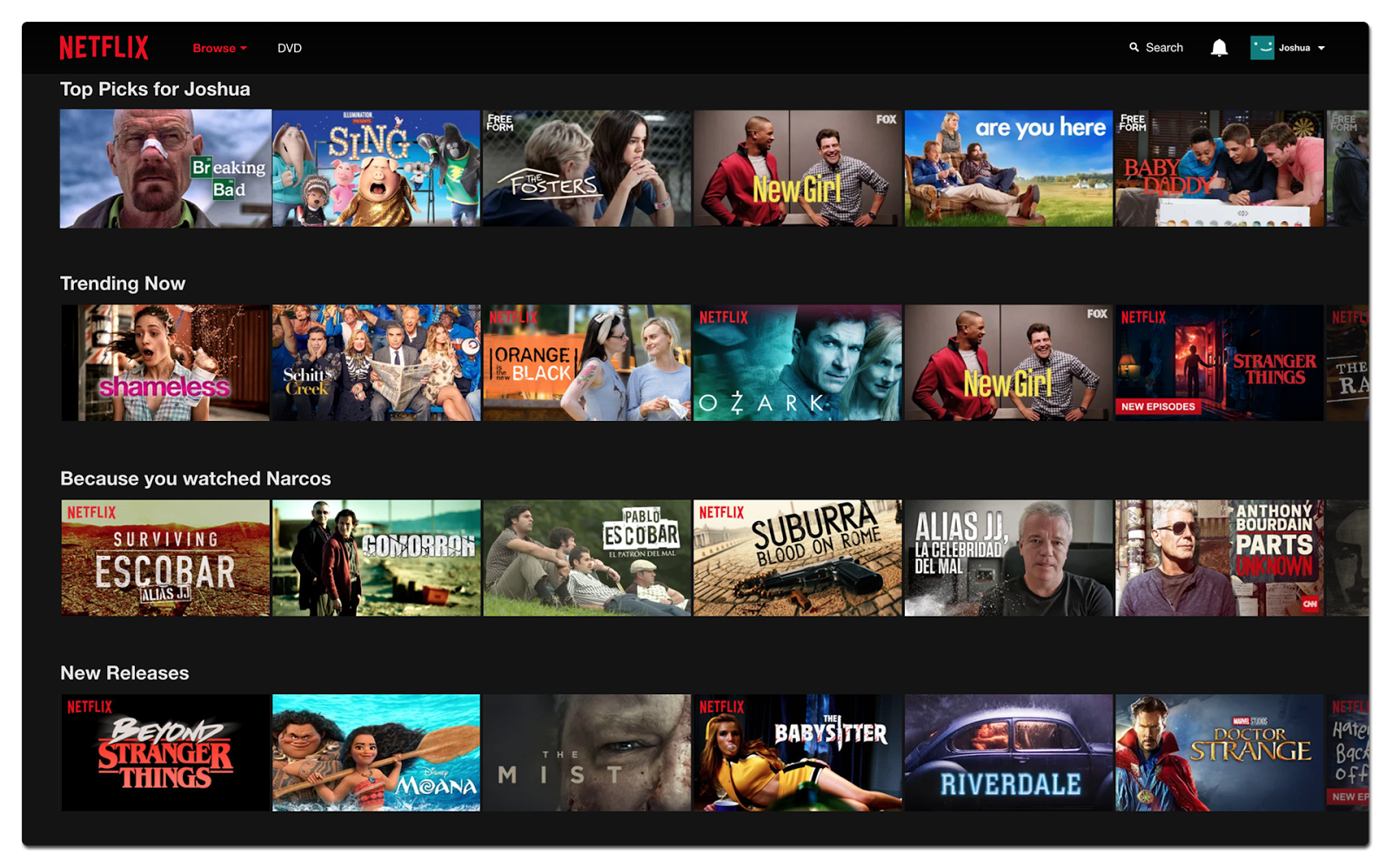
Next, let's look at the process of how a recommendation engine works.
How does an AI-based Recommendation System Work? A Four-Step Process
A recommendation system makes use of Recommender as a Service functionality, which factors in important user information to successfully predict the rating a user might give the product. It uses algorithms, data analysis, and data filtering techniques in a contextual format to understand how a specific customer might engage with and review the brand. By extension, this superpower allows marketers to maximize conversions and optimize the user experience.
Data goes through the following phases in a recommender system:
Step 1: Data Collection
Implicit and explicit data is collected--from user ratings and product comments to page views and order history, to name a few.
Step 2: Data Storage
The data is stored in different types of storage systems, such as the NoSQL database, a standard SQL database, or object storage, depending on the kind of recommendations you wish to roll out.
Step 3: Data Analysis
The recommender system analyzes the stored data via various data analysis methods such as batch analysis, real-time analysis, or near-real-time system analysis. The end goal is to find products with similar user engagement to ensure reliability and accuracy.
Step 4: Data Filtering
The data is filtered to get relevant, customized, and accurate information, which is then recommended to the user. To enable data filtering, choosing the right algorithm mentioned in the previous section is key.
Top-5 Challenges & Benefits of a Recommendation Engine
Google uses recommendation engines as an internal productivity platform to nudge employees towards the best decision options.
In this section, we'll look at the top challenges and benefits of using a recommendation system:
Synonymy: When a product/item is represented with two or more different names (for instance, romantic movie or romantic film) but has a similar meaning, the recommendation system cannot recognize whether the phrases show various items or the same item.
Increased ROI: Recommender engines can boost sales, the average cart value, and increase your revenues.
Scalability: Scaling algorithms can become challenging due to the multiplicity of products and users.
Enhanced CX: Since the recommendations are highly customized and relevant to the user, the customer experience becomes positive, leading to happier customers.
Latency: This happens when new products are added more frequently to the database. Since these items are not rated yet, the engine is unable to recommend the newer products.
Customized content: The ability to deliver customized and relevant content builds on customer loyalty and augments the brand's value.
Privacy: Issues of privacy and security can prevent users from adding personal information into the recommender system.
Boosts traffic: This tool allows brands to target users with targeted emails and personalized messaging, thereby boosting traffic to the app/website/other platforms.
Sparsity: When users do not give ratings or reviews to the products they used/purchased, finding a set of customers with similar ratings or interests becomes an issue.
Boosts retention: Since users leverage a consistent brand experience across different channels, the brand's retention rates become stable, and growth happens organically.
In Conclusion: Supercharge Your Growth with AI Recommendation Engines
Embrace a change in mindset and view recommender systems as beyond a one-dimensional marketing and sales tool. When used correctly, this all-purpose technology can recommend the right products to the T, build brand loyalty, augment customer lifetime value, and profitably remodel your customer's behavior. Don't you think so?
Want to know more about recommendation engines and how they can enhance your eCommerce store's conversion? Talk to us!
FAQs on Recommendation Engines
What is a recommendation engine?
A recommender system classifies information based on different user data points. It uses information-based filtering to identify top products depending on relevance matching and tries to predict which products the users will prefer in the long run.
Why does recommendation engine matter?
Recommendation engines can improve engagement by predicting products that customers are most likely to find relevant. A recommendation engine can improve the level of personalization in your eCommerce store and ensure that you can gain a competitive edge in the market based on personalization.
How recommendation engines can help in eCommerce growth?
Recommendation engines can boost eCommerce growth by displaying highly personalized product recommendations on different pages and boosting conversion rate for eCommerce stores.